서 론
식육 시장의 규모는 지속적으로 증가하고 있다(Godfray et al., 2018; Shahbandeh, 2023). 시장이 성장하면서 고기와 육제품에 대한 소비자의 관심이 높아지고, 원산지를 바꾸거나 유통 기한을 위조하는 부정육에 대한 문제도 많이 발생된다(Abbas et al., 2018). 식육은 단백질뿐만 아니라 지질, 무기물, 탄수화물, 비타민 등 다양한 영양원을 함유하고 있는 식품으로 미생물에 의해 쉽게 변질되거나 부패된다(Ercolini et al., 2006; Oh et al., 2016). 따라서 부정육의 유통은 경제적 문제를 야기할 뿐만 아니라 소비자들의 건강에 부정적인 영향을 미칠 수 있다(Abbas et al., 2018, Ballin, 2010). 그러므로 육류 및 육제품의 안정성을 확보하기 위해서 이러한 부정육의 유통을 근절해야 하는데, 유통과정에서 신속하고 정확하게 부정육을 판별하거나, 유통업체를 직접 단속하는 방법이 있다.
소비자들이 고기를 구매할 때, 고기의 색이나 향과 같은 관능적 특성에 의존하여 제품의 신선도 및 품질을 판단하는 것이 일반적이다(Torrico et al., 2018; Wachholz et al., 1978). 그러나 관능적인 특성에 의존한 판별은 정확성이 낮다. 또한, 다지거나 열처리 등의 공정을 거친 식육 및 육제품은 신선도 및 품질을 판단하기가 더욱 힘들다. 따라서 과학적인 방법으로 고기의 선선도와 품질을 평가하는 기술이 필요하며, 부정육이 소비자의 식탁에 오르기 전에 신속하게 유통을 차단하기 위해서는 현장에서 간편하게 적용할 수 있는, 그리고 신속하고 정확한 결과를 얻을 수 있는 기술이 요구된다.
현재까지 보고되거나 개발된 식육 판별 기술은 주로 축종, 품종, 품질, 해동육 및 부패육의 구별에 관한 것이다(Fig. 1). 판별에 사용되는 과학적 기술은 유전자 분석, 단백질 분석, 효소 활성 및 바이오 이미지 분석 기술을 기반으로 하고 있고, 이들 대부분이 국내산과 수입산, 한우와 비한우, 신선육과 해동육, 신선육과 부패육 등을 구분하여 식육의 표시방법 등을 위반한 부정육을 판별하는데 활용될 수 있다(Gottesmann and Hamm, 1983; Kim et al., 2017; Park et al., 2021). 지금까지 다양한 식육 판별 기술이 개발되거나 보고되었음에도 불구하고, 유통 현장에서 필요로 하는 판별의 신속성과 정확성, 분석비용 절감, 분석절차의 간편성 등에서 기존 기술이 극복해야 하는 한계점이 존재한다. 따라서 본 연구에서는 지금까지 개발 또는 보고된 식육 판별 기술의 특징과 문제점을 살펴보고, 앞으로 현장에서 활용이 가능한 식육 판별기술의 요건 및 개선점에 대해 제언하고자 한다.
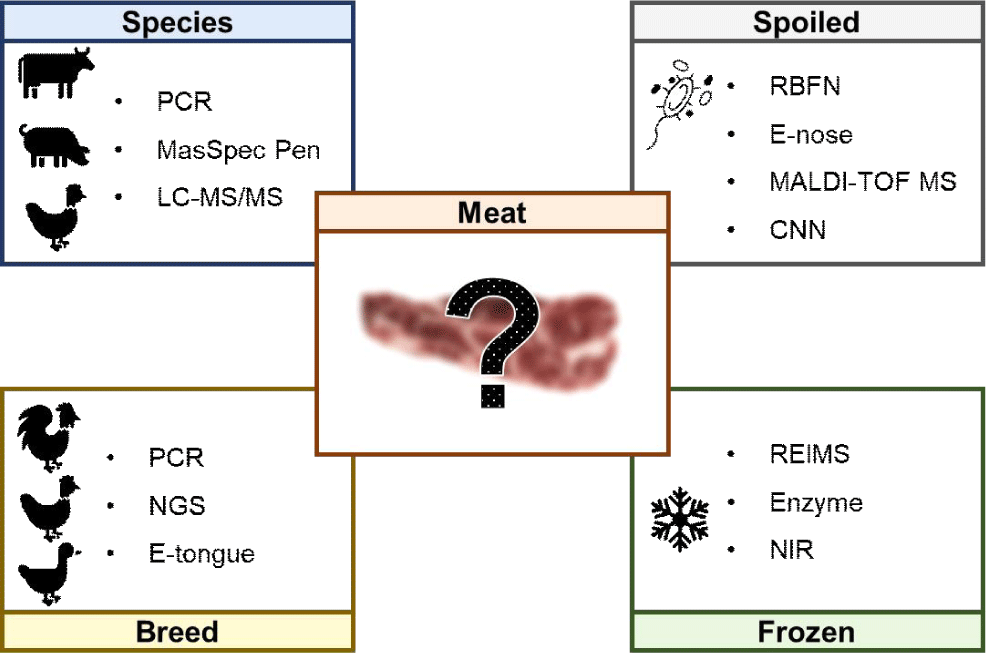
축종판별
소고기, 돼지고기, 닭고기는 사람들이 많이 소비하는 축종이다. 소비자들은 일반적으로 고기의 외향적인 요소인 육색, 조직감, 향기를 이용하여 고기를 판별한다. 그러나 이러한 방법은 고기의 부위, 절단상태, 가열상태 등의 영향을 쉽게 받으며, 이로 인해 외향적 요소로 축종을 판별하는 것은 한계가 있다.
신선육이나 육제품에서 원료의 축종을 판별을 위해 다양한 분석 기법들이 사용되고 있는데, 크로마토그래피(Lucarini et al., 2018), polymerase chain reaction(PCR; Hossain et al., 2019), near infrared(NIR), enzyme-linked immunosorbent assay(ELISA) 등이 대표적인 기법이다(Table 1). 각각의 방법은 고유의 장단점이 있으며, 기존 방법의 한계점을 극복하는 식별 기술 개발이 시도되어 왔다.
Species | Sorting type | Sorting methods | Targets | References |
---|---|---|---|---|
Bovine | Breed | PCR | Hanwoo, Holstein | Nam et al. (2004) |
Breed | NGS | Hanwoo, Holstein | Han et al. (2018) | |
Breed | SNP, NGS | Hanwoo, Ankole, N'Dama, Ogaden, Boran, Kenya Boran, Holstein, Jersey, Angus | Cho et al. (2014) | |
Meat quality | E-tongue | Angus, Buffalo, Hungarian Grey, Hungarian Spotted cattle, Hostein | Surányi et al. (2021) | |
Age | SPR | Hanwoo | Jang et al. (2014) | |
Meat cut | 2D gel electrophoresis, MALDI-TOF MS/MS | Hanwoo | Lee et al. (2010) | |
Meat grade | NIR machine learning (CNN) | Hanwoo | Seo et al. (2019) | |
Spoiled | E-nose, ANN | Beef | Panigrahi et al. (2006) | |
Frozen | REIMS | Beef | He et al. (2021) | |
Frozen | Enzyme | Hanwoo | Han et al. (2005) | |
Porcine | Breed | SNP, PCR | Iberico (bellota, cebo de campo, cebo), Berkshire, Landrace, Yorkshire, Duroc, Korean native pig, Wild pig, Spainish pic, Jeju pig | Cha et al. (2019) |
Breed meat quality | PCR | Korean native pig × Landrace × Duroc | Park et al. (2021) | |
Frozen | Enzyme | - | Toldrá et al. (1991) | |
Chicken | Breed | SNP | Korean native chicken, Broiler, laying hens | Lee et al. (2019) |
Spoiled | MALDI-TOF MS | Broiler | Höll et al. (2016) | |
Spoiled | CNN | Broiler | Putra and Prakasa (2020) | |
Frozen | NIR | Broiler | Liu et al. (2004) | |
Multiple | Species | PCR | Cattle, pig, sheep, goat, horse, deer | Park et al. (2012) |
Species | PCR | Cattle, pig, chicken, duck, sheep, goat, horse, dog, turkey, goose | Koh et al. (2010) | |
Species | NIR | Cattle, pig, chicken | Nolasco-Perez et al. (2019) | |
Species | MasSpec Pen | Cattle, pig, chicken, lamb, venison, cod, halibut, Atlantic salmon, sockeye slamon, steelhead trout | Gatmaitan et al. (2021) | |
Species | LC-MS/MS | Cattle, pig, sheep | Buckley (2016) | |
Species | LC-MS/MS | Cattle, pig, chicken, duck | Kim et al. (2017) | |
Spoiled | E-nose Random forest algorithm | - | Hanif et al. (2022) |
PCR, polymerase chain reaction; NGS, next-generation sequencing; SNP, single nucleotide polymorphism; E-tongue, electronic tongue; SPR, surface plasmon resonance; MALDI-TOF, matrix-assisted laser desorption ionization-time of flight; NIR, near infrared; CNN, convolutional neural network; E-nose, electronic nose; ANN, artificial neural network; REIMS, rapid evaporative ionization mass spectrometry; LC, liquid chromatography; MS, mass spectrometry.
PCR은 DNA를 기반으로 한 기술로 축종 판별에 가장 많이 이용되는 방법이다. 축종별 고유 유전자를 활용하여 다양한 동물 종을 구별하는데 용이한 장점이 있다. 그리고 가공 및 열처리된 제품을 분석할 수 있고, 매우 적은 샘플 양으로도 검출 정확도가 높으며 안정적인 결과를 나타낸다. 소, 돼지, 양, 염소, 말, 그리고 사슴을 PCR 기법을 활용해 간단하게 분류하는 방법이 개발된 바 있다(Park et al., 2013). 그 밖에도, 닭, 오리, 개, 칠면조, 그리고 거위에서도 DNA에 존재하는 다양한 서열을 이용하여 분석이 가능하다는 선행연구가 있었다(Koh, 2010). 하지만, 분석 과정에 DNA 추출과 증폭 과정이 필요해 분석 시간이 다소 소요되며, 가열 후에는 추출 수율이 낮아지고 그로 인해 DNA 단편화를 유발하여 분석에 정확도가 낮아지는 단점이 있다(Murray et al., 2009; Sentandreu and Sentandreu, 2014). 현재 PCR 기법은 식품의약품안전처의 식품공정에 의거하여 대립유전자 다중분석법을 시행해 한우와 비한우 여부를 판단하고 있다.
ELISA 기술은 면역형광기법을 활용한 기술로써, 항체를 이용하여 특정 축종을 분류하는 기법이다. 분석 소요 시간이 짧고, 혼합물 내 10% 정도 이상 포함되어 있는 축종을 판별할 수 있다. 그러나 단백질이 변성될 경우 식별 정확도가 낮아지며, 특히 가열과 같은 가공 공정을 거친 경우 주의를 요한다(Perestam et al., 2017).
분광광도법을 활용한 NIR 분석 방법과 partial least square-discriminant analysis(PLS-DA) 통계 기법을 활용한 돼지고기, 소고기, 그리고 양고기의 판별이 연구되었고, 최근 닭고기를 판별한 결과 또한 보고된 바 있다(López-Maestresalas et al., 2019; Nolasco-Perez et al., 2019; Rady and Adedeji, 2018). NIR을 이용한 분광광도법은 분석 시료를 파괴시키지 않고 고체 물질을 온전히 분석할 수 있다는 장점이 있는 반면, 특이성이 부족하여 정확한 분석을 위해서는 광범위한 분석이 필요하다(Zia et al., 2020).
크로마토그래피 기반 기술 또한 활용도가 높은데, GC는 주로 휘발성/휘발성 분석에 이용되고 high-performance liquid chromatography(HPLC)는 아미노산, 탄수화물, 비타민, 그리고 페놀 화합물과 같은 물질을 검출하는데 주로 이용된다(Santos and Oliveira, 2017). 선행연구에서 GC를 이용해 C20:2 지방산을 분석하여 돼지고기를 판별한 바 있지만, 이는 정어리나 고등어와 같은 생선에서도 확인된 바가 있어 신뢰도가 낮다고 할 수 있다(Mahama et al., 2020; Marikkar et al., 2016). LC-MS(liquid chromatography-mass spectrometry)를 이용한 정성적 단백질 분석을 통해서도 판별이 가능하며, 더 나아가 LC-MS/MS를 이용하면 특정 펩타이드를 분석하여 축종을 구분할 수도 있다(Chou et al., 2007; Kim et al., 2017; Sarah et al., 2016). 선행 연구들에서는 LC-MS/MS를 활용하여 닭, 돼지, 소, 양, 오리, 토끼 등 다양한 축종을 판별하는 높은 활용도를 보였지만, 소요 시간이 많을 뿐만 아니라, 운용 비용 또한 높으므로, 보다 빠르고 정확하며 저렴한 판별 기술이 필요하다(Claydon et al., 2015; Kim et al., 2017; Li et al., 2018; Stachniuk et al., 2021).
이전 기술의 단점들을 보완하고자 전자코(electronic nose, e-nose) 기술을 활용하였다. 이는 전자 센서를 이용하여 휘발성 물질을 식별하는 기술이다. 전자코 기술은 축종을 판별하는 크로마토그래피 기반 기술이나 유전자 기반 판별 기술에 비해 소요 시간이 적으나, 기준점을 LC-MS로 분석하지 않으면 사용이 어렵다는 한계점이 있다.
품종판별
국내 소고기 수입 시장이 개방되면서 한우보다 저렴한 소고기들이 수입되고 있는데, 이로 인해 수입산 소고기를 한우로 허위 판매하는 문제가 발생했다. 그리고 미국에서 광우병 발생 이후 수입 소고기에 대한 소비자들의 우려가 커졌다. 소뿐만 아니라, 우리나라 전체 육류 생산의 20% 이상을 차지하고 있는 가금류의 종자는 수입에 의존하고 있고, 돼지 또한 유사한 실정이다. 이러한 이유로 고기의 품종 판별 기술에 대한 요구가 증가하였고 현재 다양한 분석 방법을 이용해 종의 품종을 판별하고 있다(Table 1).
유전자 분석 기반 기술을 이용한 품종 구별을 하고 있는데, 소에서 DNA 마커를 이용하여 한우와 홀스테인을 구분한 바 있고(Cheong et al., 2013; Nam et al., 2006), NGS 기법을 통해서도 다양한 품종에서 한우를 판별한 바 있다(Cho et al., 2014; Han et al., 2018). 뿐만 아니라, Cha 등(2019)은 9개의 돼지 품종을 유전자 분석 기술을 이용하여 식별하였다. 돼지는 주로 잡종강세를 활용하여 육질 향상을 위해 이종교배를 하고 있다(Edwards et al., 2003). 우리나라에서는 한국재래돼지×랜드레이스×듀록을 교배하여 난축맛돈을 개량한 바 있는데 이러한 난축맛돈 유전자 내 품종 식별 및 등심 근육 성장과 발달에 관여하는 마커를 PCR 기술로 확인하고 식별해 내었다(Park et al., 2021). Lee 등(2019)의 선행연구에서는 유전자 기술인 SNP 마커를 이용하여 토종닭을 식별하였다. 축산물품질평가원에서는 DNA 동일성 검사를 통해 소와 돼지의 이력관리를 하고 있다.
품종 식별은 분자 기반으로 식별이 가능하다(Juárez et al., 2008). 닭은 불포화 지방산이 풍부하며, 이로 인해 지질 산화와 휘발성 유기 화합물이(volatile organic compounds, VOC) 쉽게 발생한다(Mancinelli et al., 2021). 또한 지방구성은 닭의 품종에 따라 달라지며 이를 이용하여 LC-MS 기반으로 닭의 복부 지방의 지질분석을 통해 닭의 품종을 식별이 가능하다(Li et al., 2022). 한국특허공개 제10-2012-0009974에서는 GC/MS를 이용하여 휘발성 화합물 프로파일링을 통해 토종닭과 수입닭을 판별하는 기술이 개시되었으며, 더 나아가 이 기술은 계육외 우육, 돈육, 오리육, 칠면조육, 염소육, 양육, 말육 또는 구육 등 식별에도 용이할 수 있다. 국내산 돼지일 경우 백신접종을 통해 돼지열병 항체를 보유하고 있기 때문에 면역크로마토그래피 기술을 통해 돼지열병의 항원을 이용하여 국내산과 수입산 돼지고기를 판별할 수 있다(Jung et al., 2021). 이 기술을 이용하여 국립농산물품질관리원에서는 국내산 돼지고기와 수입산 돼지고기를 유통 현장에서 신속하게 판별하고 있다.
대부분의 판별 기술은 분석 전 다양한 물리적, 이화학적 처리가 가해진다. 이에 반해, 분광법은 주로 샘플에 화학적 처리가 가해지지 않고 분석이 가능하다. 근적외선 분광법(NIR)은 별도의 시약이 필요하지 않으며 폐기물도 생성하지 않는 비파괴적인 기술로써, 스캔된 시료에서 분자결합과 미세구조를 파악한다(Downey and Hildrum, 2004). NIR를 통해 듀록과 이베리코 품종을 판별하였다(del Moral et al., 2009). 그 외에도, HRMAS-NMR은 고분해능 매직앵글스핀-핵자기공명을 활용한 기술의 이용으로, 소의 대사 프로파일링을 통하여 버팔로와 키아니나를 정확도 높게 식별하였지만, 홀스테인은 정확도 높게 식별하지 못하였다(Ritota et al., 2012). 분광법은 시료 분석을 비파괴적으로 진행하는 장점이 있으나, 판별 정확도가 품종에 따라 많이 다르며 고도의 데이터 분석이 별도로 필요하다는 어려움이 있다.
육질판별
육류 산업이 국내외로 증가함에 따라 소비자들의 육류 품질에 대한 관심도 증가하게 되었다. 소비자들의 만족도에 영향을 미치는 대표적인 요소는 맛, 조직감, 다즙성이다(Cheng and Sun, 2008; Maltin et al., 2003; Shahidi et al., 1986). 고기의 맛과 구성성분은 축종, 품종, 성별, 사료의 영향을 받는다(Uhliřova et al., 2017; Mushi et al., 2008; Melton, 1990). 근내 지방은 풍미, 육즙, 연도를 향상시키는 요인으로 육질에서 중요한 요소이다(Hocquette et al., 2010).
소비자들은 합리적인 가격과 고품질의 제품을 요구하게 되었고, 이를 충족시키기 위하여 식육의 품질 평가 기술은 육류 산업 내에서도 중요한 사항 중 하나이다. 일반적으로, 육질을 평가하는 항목으로는 pH, 육색, 연도, 보수력, 조지방 혹은 마블링 등이 이용된다. 특히, pH와 육질은 매우 밀접한 관계가 있다. pH가 높으면 육색이 어두워지며, 육즙 손실이 적어지게 된다. 이로 인해 육즙이나 고기의 부드러움에 영향을 미친다(Berri et al., 2019).
식육의 품질을 분석 시 각각 항목에 따라 다양한 이화학적 분석 기법과 관능분석이 사용된다. 관능분석은 고도로 훈련된 숙련자들에 의해 이뤄져야 하며, 결과는 다소 주관적이며 평가자들에 따라 달라지기 쉽다.
대사체학 혹은 지질체학과 같은 소분자를 측정하는 기술은 육류의 품질 특성을 측정하는데 활용이 된다. 단백질체학(proteomics)을 이용하여 단백질 바이오마커를 선별하여 고기의 품질 특성인 연도와 육색, 보수력, pH 예측을 시도하고 있다(Di Luca et al., 2016; Gagaoua et al., 2018; Gagaoua et al., 2021). 그러나 이와 같은 분석 기술들은 분석 절차가 복잡하고 비용이 많이 발생하며, 분석 시간이 또한 많이 소요된다. 이에 소비자들이 육류와 육제품을 구매하기 전에 가격이 합리적인지에 대한 신속한 판별 기술이 요해진다.
식육의 품질을 평가하는 기술로 분광학 기반 기술이 소개되었다(Monin, 1998). 분광학 기반 기술을 통하여 육류 내 화학 물질을 측정하여 육질 특성 예측이 가능하다(Alomar et al., 2003). 근적외선분광법(near-infrared spectroscopy, NIRS)을 이용하여 소고기에서 육색을 측정하였지만 보수력과 pH은 직접적으로 측정하지 못하였다(Prieto et al., 2008). 반면에 Andrés 등(2008)은 분광학 기반 기술을 이용하여 소고기의 육색과 pH을 사후 24시간에 높은 수준으로 예측하였다.
최근에는, 분석 시간을 단축하는 기술로 전자 감각 센서를 활용한 기술이 소개되었다. 전자코(E-nose)는 생물학적 후각을 모방하여 화학센서를 통해 냄새를 감지하고 구별해 내는 기기로써, 분석 시 시간이 적게 소요되며 샘플을 비파괴적으로 분석이 가능하다(Jia et al., 2018; Loutfi et al., 2015). 전자혀(E-tongue)는 전자코와 비슷하게 감각 센서를 이용하여 품질을 분석하는 기술로, 5종류의 소 품종(앵거스, 버팔로, 헝가리 회색소, 헝가리 얼룩소, 홀스타인)에서 pH, 수분활성도, 건물 함량을 높은 정확도로 예측하였다(Surányi et al., 2021).
전자 감각 센서를 활용한 기술은 센서를 활용한 기술로 샘플을 비파괴적으로 분석가능하며 시간소요가 적게 발생한다. 하지만, 개발 시 기존에 이용되는 대사체학 혹은 지질체학과 같은 소분자 분석 결과와 대조가 필요하며 분석 시 외부환경에 영향을 많이 받아 주의가 필요하다.
냉장육 또는 해동육 판별
고기를 냉동시 고기 내에 수많은 얼음 결정이 생기게 되며 이로 인해 조직의 미세구조 파괴되고 구조가 변한다. 얼음 결정은 동결 속도, 압력, 동결 시간에 따라 달라진다(Martino and Zaritzky, 1988). 느리게 동결하면 큰 얼음 결정이 생기는 반면에 빠르게 동결하면 작은 얼음결정들이 고기 조직에 생긴다(Behnke et al., 1973; Martino et al., 1998; Sanz et al., 1999). 얼음 결정의 크기는 고기내 구조 파괴의 정도와 연관이 있다.
냉동하지 않은 돼지고기와 냉동한 돼지고기를 비교하였을 때 냉동 시 근섬유 다발 파괴가 크게 일어난다(Martino et al., 1998; Molina-Garcı́a et al., 2004; Zhu et al., 2004). 이는 소고기에서도 동결시에 조직 파괴가 증가한다고 보고된 바 있고, 미세 구조에서도 확인된 바 있다(Martino and Zaritzky, 1988).
냉장육과 냉해동육을 판별하는 주요 기술은 효소분석법이다. 대표적으로 미토콘드리아에 존재하는 β-hydroxyacyl-CoA-dehydrogenase method(HADH)를 측정하는 방법이 있다(Gottesmann and Hamm, 1983). 고기를 동결-해동할 경우 미토콘드리아와 리소좀의 파괴로 인해 효소를 분비한다. 하지만, 미토콘드리아 파괴는 고기를 다질 경우에도 발생한다. 또한, 효소는 시간이 지남에 따라 고기내의 단백질 분해 효소로 인해 영향을 받는다. 그렇기 때문에 효소분석법 외에도 동결육 판별 기술이 필요하다.
동결로 인한 파괴는 미토콘드리아 외에도 단백질 파괴 및 변성에 영향을 미쳐 마이오글로빈 결합 형태에도 영향을 준다(Jeong et al., 2011; Wagner and Añon, 1985). 육색은 마이오글로빈의 상태에 의해 변화한다. NIR 및 NMR은 분광분석법의 일종으로 냉장육과 냉동육을 판별하는데 이용하는 기술로 사용이 가능하다. 닭고기를 4℃, 0℃, –3℃, –12℃, –18℃ 보관 후 냉동-해동하였을 때, two-dimensional visible은 445, 475, 560 mm 파장에서 deoxymyoglobin, metmyoglobin, oxymyoglobin과 연관이 있게 나타났다(Liu and Chen, 2000; Liu et al., 2004).
육색 외에도 동결로 인한 구조의 파괴는 고기의 보수력이 나빠지게 한다. 냉장육보다 냉해동육이 소고기, 돼지고기에서 높은 육즙감량이 발생한다(Hansen et al., 2003; Ramsbottom and Koonz, 1939).
DNA는 사후에 엔도뉴클레아제, 엑소뉴클레아제, 가수분해, 산화, 알킬화에 의해 분해된다. 고기를 동결할 경우 DNA 파괴가 증가한다. Neutral sucrose gradient centrifugation assay 방법을 이용하여 DNA 파괴를 측정하였을 때, –16℃에서 12개월 동안 동결보관 시 Escherichia coli는 dsDNA의 파괴가 증가하였다(Grecz et al., 1980). 냉장 소고기와 냉동 소고기 DNA 손상을 comet assay를 이용하여 비교하였을 때 동결할 경우 DNA가 파괴되는 정도와 양의 상관관계를 가지고 있다(Park et al., 2000). 하지만 활용되고 있는 방법은 대부분 불특정한 시료를 분석할 때, 비교를 위한 대조군이 필요하다.
부패육 판별
육류는 단백질 및 기타 필수 영양소 공급원으로 이용되는 대표적인 식품이다. 하지만, 부패성 미생물과 병원성 미생물의 성장에도 좋은 공급원으로 이용될 수 있다. 식육의 부패는 미생물의 성장과 효소 활동을 통하여 분해 및 대사산물 형성하는 것으로, 이취, 악취, 변색, 가스발생, 물리적 혹은 화학적 형태 변화가 발생한다(Katiyo et al., 2020).
주요 부패 미생물로는 슈도모나스(Pseudomonas) 속의 호기성 저장 육류의 부패균인 Moraxella spp., Ancinetobacterspp.가 알려져 있다(Casaburi et al., 2015; Ercolini et al., 2010; Labadie, 1999). 육류의 미생물은 가공방법, 운송, 보관방법에 의하여 달라지며 이는 초기 고기 미생물의 종류에 따라 우점 미생물에 영향을 주기 때문이다(Nychas et al., 2008). 우리나라에서는 식품의약품안전처고시 제2018-2호에 의해 소고기, 양고기, 돼지고기, 닭고기 오리고기는 미생물 검사 결과 일반세균수가 5×106(CFU/g, CFU/cm2) 이하여야 하며, 대장균수는 1×103(CFU/g, CFU/cm2) 이하로 기준을 정하고 있다. 부패육 검사는 검역원고시에 따라 평판배양법이나 건조필름법을 이용하여 세균수를 측정하는 방법이 사용된다.
식품의 부패를 식별하기 위해서는 부패를 시키고 있는 미생물군을 식별하는 방법을 사용할 수 있다. 미생물 식별에 가장 널리 알려진 기술은 16S rRNA 유전자 앰플리콘 시퀀싱을 이용하여 식별하는 방법이다(Połka et al., 2015). 하지만 이는 미생물의 과 혹은 속만 식별이 되며 하위 단계의 식별에는 제한적이다. MALDI-TOF MS는 저분자량 하위 프로테옴으로 박테리아를 식별할 수 있으며, 신속하게 분석이 가능하다(Holland et al., 1996; Seng et al., 2009). 또한 생물형이나 계통 수준에서 구별이 가능하다. 하지만 미생물 식별 기술은 고가의 장비가 필요하여 많은 비용이 발생한다. 이 외에도 육류의 부패를 측정하기위한 이화학적 방법과 미생물학적 방법뿐만 아니라 크로마토그래피, 분광광도법, E-nose, 인공지능 기술을 통한 검출 방법이 있다.
생체아민(biogenic amine)은 고기와 같이 단백질 함량인 높은 식품에서 많이 생성되며, 주로 미생물 효소 활동으로 인하여 특정 아미노산이 탈카르복실화 결과로 형성된다(Halász et al., 1994). 크로마토그래피 기술을 통해 생체아민을 측정하여 식육의 부패를 판별하였다. 아민 생성은 미생물 활동으로 생성될 뿐만 아니라, 식육내 효소작용으로 인하여 생성된다. 그리고 적색육과 백색육에 따라 생성정도가 달라진다(Vinci and Antonelli, 2002). 액체크로마토그래피(HPLC)를 통하여 높은 정확도로 아민을 부패지표로 식별하였다(Vinci and Antonelli, 2002). 그러나 크로마토그래피 식별 방법들은 식육의 품질 및 유통기한을 예측하기 위한 지표를 정량화 하기는 좋으나 고가의 장비가 필요하며 분석시간이 많이 소요된다. 따라서 식품 가공기술의 발전 및 다양화에 대한 식육의 품질 및 유통기한을 예측하기 위한 신속한 분석법이 필요하다.
분광법 분석은 비파괴적이며 샘플 전처리가 비교적 최소한으로 필요하며 특정 소모품이나 시약이 필요하지 않은 장점이 있다. 푸리에변환적외선분광법(Fourier-transform infrared spectroscopy, FTIR)을 이용하여 돼지고기 부패와 미생물 그룹간의 상관관계가 관찰되었다(Papadopoulou et al., 2011). 다중 스펙트럼 이미징 시스템(multispectral imaging system)을 이용하여 돼지고기의 부패를 76.13%의 정확도로 식별해 내었다(Dissing et al., 2013). 분광법 기반 기술은 분석 시간이 적은 장점이 있으나 정확도가 크로마토그래피 기반 기술에 비해 낮으며 미생물 종류에 따른 분석 결과가 달라질 수 있다.
현장에서 빠르게 이용될 수 있는 기술로 전자코가 활용 가능성을 보였다. 전자코를 활용하여 식품의 부패 시 발생하는 대사산물(휘발성 유기 화합물)을 감지하여 고기에서 부패를 측정하였다(Andre et al., 2022; Panigrahi et al., 2006; Wojnowski et al., 2017). 전자코를 통한 결과신호는 복잡하므로 주성분분석(principal component analysis, PCA), 부분최소제곱분석(partial least squares, PLS)와 같은 고도의 분석과정이 필요하다. 그리고 탐지 기능을 확인하기 위해서는 독립적으로 식육의 부패에 대한 연구가 요구된다. 최근에는, 인간의 신경망을 모방한 인공신경망 기술(artificial neural network, ANN)을 활용하여 전자코 분석 결과를 통해 부패된 소고기를 식별해 내었다(Panigrahi et al., 2006). Hanif 등(2022)은 Random Forest 알고리즘과 전자코 결과를 이용하여 미생물 개체수 예측하였다. 하지만 센서 기반 기술은 환경에 영향을 받기 쉬우며 정량 분석에 한계가 있다.
연구동향
최근에는 기존의 기술과 첨단 분석 기술을 접목하여 좀더 정확하고 신속하게 분석하는 방법이 연구되고 있다. 식육을 분석하기 위해 PCR(Hossain et al., 2019; Park et al., 2013), ELISA(Perestam et al., 2017), 분광법(del Moral et al., 2009; Monin, 1998), 크로마토그래피(Lucarini et al., 2018) 등 다양한 기술들이 이용된다. 그중 분광법은 식육을 판별할 때 신속하고 비파괴적으로 식별할 수 있는 장점이 있다. 이 기술은 육질 평가, 구성성분 분석, 부위, 변질 및 부패 여부를 판단하는 데 이용되고 있다(Bai et al., 2024; Prieto et al., 2009; Zhu et al., 2021). 그러나 분광법으로만 판별 시 정확도가 다소 낮은 단점이 있다. 이러한 단점을 극복하는 방법으로 기존의 분광법 기술인 근적외선 분광법(NIRS)과 초분광 이미징(hyper-spectral imaging, HIS)을 융합하는 기술이 보고되었는데, 신선도 판별의 경우 92.6%의 높은 정확도가 확인된 바가 있다(Zhu et al., 2021).
최근에는 인공지능 기술(artificial intelligence, AI)을 활용한 식육 판별법이 보고되었다(Zhang et al., 2022). 즉, VIS/NIR 분광 데이터와 인공 신경망(ANN)과 같은 인공지능 기술을 이용하여 돼지고기의 품질 특성을 기존의 기술보다 더 높은 정확도로 예측하였다(Tang et al., 2023).
한편, 센서를 이용한 전자코 및 전자혀를 활용한 연구도 많이 이뤄지고 있다. 식육이 변질 또는 부패되는 과정에서 생성되는 다양한 휘발성 물질들을 정성 분석하여 신선도를 판별하는 기술이 보고되었다(Andre et al., 2022; Zaytsev et al., 2024). 그러나, 센서를 이용할 경우 주위 환경에 영향을 받을 수 있으며, 정량 분석이 어려운 단점이 있다. 식육의 신선도 측정에 멀티센서를 이용하여 단일 센서의 오류를 최소화하고 딥러닝(심층신경망) 기술을 적용하여 판별 정확도를 90%으로 높일 수 있다고 보고되기도 했다(Jang et al., 2020). 이와 같이 최근 연구 동향은 기존의 분석 및 판별 기술을 보다 정확하고 신속하게 분석해낼 수 있는 방안에 대하여 연구되고 있다.
결 론
식육 시장이 지속적인 성장에 따라 다양한 기술들이 육류를 식별하는데 이용되고 있다. 부정육은 육류 산업의 경제적 손해 외에도 소비자의 건강과 인식에도 영향을 미친다. 이에 식육 판별 기술은 중요하다. 기존의 기술들은 노동 집약적이거나 고가의 장비가 필요한 기술들이 대부분이다.
분석 시간소요를 단축시키기 위한 다양한 기술들이 활용되고 있으며 대표적으로 분광학 기반 기술이나 감각센서를 활용한 기술이 활용되고 있다. 하지만 이러한 분석기술 또한 대부분 현장에서 이용만 가능하고 소비자들이 쉽게 접근하기 어렵다.
본 연구에서는 현재 육류 식품에 활용되고 있는 축종, 품종, 육질, 그리고 부패의 부정육 판별 기술의 현황과 문제점을 파악하였다. 이를 종합해보면, 현재의 판별 기술들은 정확하지만 파괴적이고 소요 시간이 길고, 혹은 빠르고 편리하지만 정확도가 떨어지는 한계가 있다. 그리고 이들은 업계에서의 판별에 주로 활용되고 있으나, 이용자는 시험 기관이나 감독 기관으로 극히 한정적이다.
현장에서는 국내산 돼지고기와 수입산 돼지고기를 식별하기 위해 면역크로마토그래피 기술을 기반으로 한 키트를 제작하여 신속하게 판별하고 있다. 이와 같이 식육의 축종, 품종, 품질 및 각종 특성을 신속하게 구별해낼 수 있는 마커들을 발굴하여 신속하고 정확도 높은 판별 또는 검사 키트를 개발하는 것이 필요하다. 또한 육류 시장에서 소비단계에서도 소비자들이 직접 식육을 판별하는 기술 개발도 필요할 것이다.